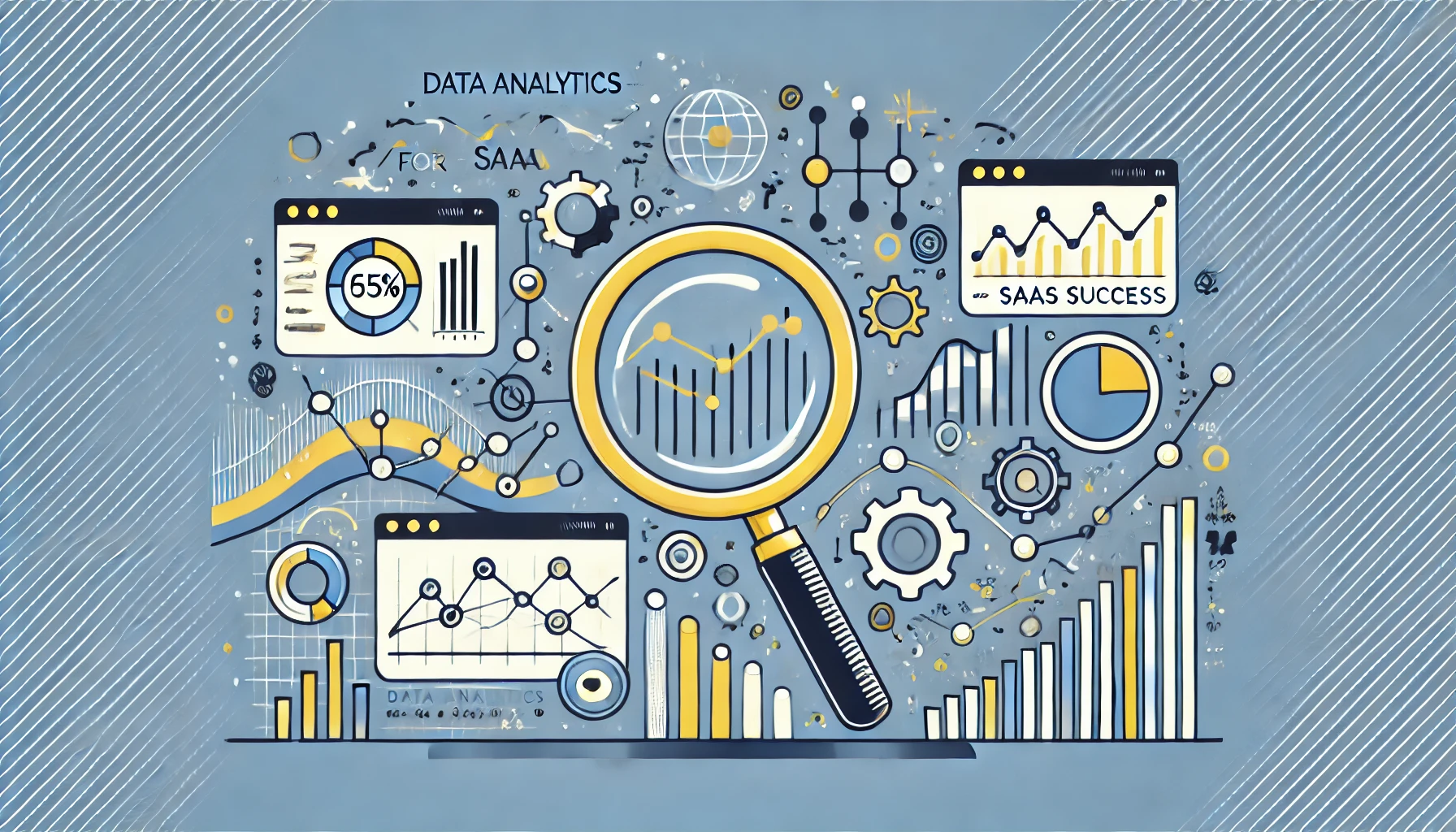
To thrive, SaaS businesses need more than just innovative software—they need the ability to make informed decisions based on real-time data. This is where data analytics comes into play. Leveraging data analytics for SaaS success isn’t just a competitive advantage; it’s a necessity.
- 74% of SaaS companies report that data analytics directly contributes to better decision-making.
- Companies using data-driven approaches are 23 times more likely to acquire customers.
- SaaS businesses that use predictive analytics see a 50% improvement in customer retention.
By understanding and acting on key metrics, implementing a data-driven culture, and utilizing the best SaaS analytics tools, companies can unlock growth, optimize pricing, and enhance user experience.
In this comprehensive guide, we’ll explore how SaaS companies can harness the power of data analytics to drive success.
What Are the Key Metrics SaaS Companies Should Track?
Understanding which metrics to track is the foundation of effective data analytics in the SaaS industry. These metrics provide insights into the health of your business, customer satisfaction, and overall growth potential. Let’s break down some of the most critical metrics SaaS companies should monitor.
1. Customer Acquisition Cost (CAC)
Customer Acquisition Cost (CAC) measures the total cost of acquiring a new customer, including marketing, sales, and onboarding expenses. It’s a key metric because it directly impacts profitability. Lowering CAC while maintaining or improving customer acquisition rates is a common goal for SaaS companies. By analyzing CAC, businesses can identify which channels are most effective and allocate resources more efficiently.
2. Customer Lifetime Value (CLTV or LTV)
Customer Lifetime Value (CLTV or LTV) estimates the total revenue a company can expect from a single customer over the duration of their relationship. CLTV is crucial for understanding the long-term value of customers and ensuring that the cost of acquiring them is justified. A high CLTV compared to CAC indicates a sustainable business model.
3. Churn Rate
Churn rate is the percentage of customers who cancel their subscriptions within a given period. High churn rates can be a red flag, signaling dissatisfaction with the product, pricing, or support. By analyzing churn data, SaaS companies can identify patterns and implement strategies to improve retention.
4. Monthly Recurring Revenue (MRR) and Annual Recurring Revenue (ARR)
MRR and ARR are the lifeblood of any SaaS business, representing the predictable revenue generated from subscriptions on a monthly or yearly basis. Tracking MRR and ARR helps companies gauge growth, forecast future revenue, and make informed decisions about scaling.
5. Net Promoter Score (NPS)
Net Promoter Score (NPS) is a customer satisfaction metric that measures the likelihood of customers recommending your product to others. A high NPS indicates strong customer loyalty and satisfaction, while a low score suggests areas for improvement. Regularly monitoring NPS can help SaaS companies stay attuned to customer needs and preferences.
6. Customer Engagement Metrics
Engagement metrics such as active users, feature usage, and session duration provide insights into how customers interact with your product. High engagement typically correlates with higher retention and customer satisfaction. By analyzing these metrics, SaaS companies can identify which features drive value and which may need enhancement.
7. Revenue Churn
Revenue churn is slightly different from customer churn—it measures the amount of revenue lost due to customer cancellations or downgrades. This metric is critical because it directly affects the bottom line. By focusing on reducing revenue churn, SaaS companies can maintain a steady growth trajectory.
8. Customer Onboarding Success Rate
The onboarding process is crucial for customer retention. A smooth onboarding experience leads to quicker adoption and higher satisfaction. Tracking the success rate of onboarding—how quickly and effectively customers start using the product—can help SaaS companies refine their onboarding strategies and improve overall customer experience.
For example, if a SaaS company notices a high churn rate through their SaaS data analytics, they might investigate user feedback to uncover product issues or onboarding challenges. This data-driven approach enables targeted improvements that can reduce churn and enhance customer retention.
How Can SaaS Companies Implement a Data-Driven Culture?
Building a data-driven culture within a SaaS company is essential for maximizing the benefits of data analytics. A data-driven culture means that decisions at all levels are based on data, not just intuition or experience. Here’s how SaaS companies can foster this culture.
1. Leadership Buy-In and Advocacy
For a data-driven culture to take root, it must start at the top. Leadership needs to champion the importance of data in decision-making and lead by example. This means using data to inform strategic decisions and encouraging teams to do the same. When executives prioritize data, the rest of the organization is more likely to follow suit.
2. Data Accessibility and Democratization
Data should be accessible to everyone in the organization, not just data scientists or analysts. This can be achieved by implementing user-friendly SaaS analytics tools that allow non-technical team members to access and interpret data. When data is democratized, teams can make informed decisions quickly and independently.
3. Training and Education
Investing in data literacy training for employees is crucial. This training should cover the basics of data analysis, how to use analytics tools, and how to interpret key metrics. When employees are comfortable working with data, they are more likely to incorporate it into their daily decision-making processes.
4. Setting Clear Data Goals
To ensure that data is being used effectively, companies should set clear, measurable goals for their data initiatives. These goals might include improving specific metrics (e.g., reducing churn by a certain percentage) or achieving broader objectives (e.g., fostering a more data-driven decision-making process across departments).
5. Fostering Collaboration Across Teams
Data-driven decision-making should not be siloed within individual departments. Encouraging collaboration between teams—such as marketing, sales, product development, and customer success—ensures that data is shared and used holistically. Cross-functional teams can work together to analyze data from different angles and develop more comprehensive strategies.
6. Incentivizing Data-Driven Decisions
Incentivizing data-driven decisions can help reinforce this culture. For example, rewarding teams or individuals who use data to achieve significant business outcomes can motivate others to follow suit. Whether through recognition, bonuses, or other incentives, celebrating data-driven successes can reinforce the importance of data within the company.
7. Regularly Reviewing and Refining Data Practices
A data-driven culture is not static; it requires ongoing review and refinement. Companies should regularly assess how data is being used, identify any gaps or challenges, and make adjustments as needed. This iterative process ensures that the organization remains agile and continues to leverage data effectively as it grows.
For instance, a SaaS company could implement weekly “data huddles” where teams review the latest SaaS metrics and discuss how these insights could inform their current projects. By making data analysis a regular part of the workflow, companies can gradually shift towards a more data-centric culture.
What Are the Best Tools for SaaS Data Analytics?
Choosing the right tools is critical for effectively leveraging data analytics in a SaaS business. The right SaaS analytics tools can help companies collect, analyze, and visualize data to make informed decisions. Here are some of the top tools that SaaS companies should consider.
1. Google Analytics
Google Analytics is a powerful and widely used tool that provides insights into website traffic, user behavior, and conversion metrics. For SaaS companies, it can be particularly useful for tracking the performance of marketing campaigns, understanding user journeys, and identifying areas for improvement in the conversion funnel.
2. Mixpanel
Mixpanel is an advanced product analytics tool that focuses on user engagement and behavior. It allows SaaS companies to track specific actions within their product, such as feature usage, sign-ups, and in-app purchases. Mixpanel’s cohort analysis and funnel reports help businesses understand how different segments of users interact with the product over time.
3. Amplitude
Amplitude is another product analytics platform that excels in tracking user behavior and product usage. It offers real-time data analysis, advanced segmentation, and behavioral cohorts, making it ideal for SaaS companies looking to dive deep into user interactions. Amplitude’s insights can help product teams prioritize features and optimize the user experience.
4. Tableau
Tableau is a leading data visualization tool that allows companies to create interactive, shareable dashboards. For SaaS businesses, Tableau can be used to visualize complex data sets and make them more accessible to non-technical stakeholders. Its drag-and-drop interface and integration capabilities make it a favorite for many data teams.
5. Looker
Looker is a data exploration and business intelligence tool that enables SaaS companies to create custom reports and dashboards. Its powerful data modeling layer allows users to define metrics and dimensions in a consistent way, ensuring that everyone in the organization is working with the same data definitions. Looker is particularly valuable for companies with complex data needs.
6. Segment
Segment is a customer data platform that helps SaaS companies collect, unify, and activate customer data across different tools. It simplifies the process of gathering data from various sources—such as websites, mobile apps, and CRM systems—and sending it to analytics tools like Google Analytics or Mixpanel. Segment is essential for creating a unified view of the customer journey.
7. Heap
Heap is an automatic data capture tool that records all user interactions with a product without the need for manual event tracking. This makes it incredibly easy to get started with analytics, as you can retroactively analyze data without having to predefine events. Heap is particularly useful for SaaS companies that want to quickly identify user behavior patterns and optimize their product.
8. Kissmetrics
Kissmetrics is a customer analytics platform that focuses on tracking individual user behavior across multiple devices and sessions. It’s particularly valuable for SaaS companies that want to understand customer journeys, from acquisition to conversion and beyond. Kissmetrics provides insights into how different marketing channels and campaigns influence user behavior.
9. Datadog
Datadog is a monitoring and analytics platform for cloud-based applications. While it’s primarily used for infrastructure monitoring, Datadog also offers powerful tools for tracking application performance, user experience, and business metrics. For SaaS companies with complex cloud environments, Datadog provides comprehensive visibility into system performance and user interactions.
10. ChartMogul
ChartMogul is a subscription analytics platform designed specifically for SaaS companies. It provides insights into key metrics like MRR, ARR, churn rate, and CLTV. ChartMogul’s powerful integrations with billing systems make it easy to track subscription metrics in real-time and identify trends that impact growth.
How Can SaaS Companies Use Data to Improve User Experience?
User experience (UX) is a critical factor in the success of any SaaS product. A seamless, intuitive, and enjoyable user experience leads to higher customer satisfaction, increased engagement, and lower churn rates. Here’s how SaaS companies can leverage data analytics to enhance UX.
1. Analyzing User Behavior
One of the most effective ways to improve UX is by analyzing user behavior data. By tracking how users interact with the product—such as which features they use most, where they drop off, and how long they spend on each task—SaaS companies can identify pain points and areas for improvement. Tools like Mixpanel and Amplitude are particularly useful for this type of analysis.
2. Personalizing the User Experience
Personalization is key to creating a user experience that resonates with customers. By leveraging data on user preferences, behaviors, and past interactions, SaaS companies can tailor the product experience to individual users. For example, personalized onboarding flows, feature recommendations, and targeted content can make the product more relevant and engaging.
3. A/B Testing and Experimentation
A/B testing is a powerful method for optimizing UX by comparing different versions of a product feature or interface. By using data to determine which version performs better in terms of user engagement, satisfaction, or conversion rates, SaaS companies can make informed decisions about product design and functionality. Regular experimentation and testing ensure that the product evolves based on user feedback.
4. Gathering and Analyzing User Feedback
User feedback is invaluable for understanding the strengths and weaknesses of a product’s UX. SaaS companies should regularly collect feedback through surveys, interviews, and in-app prompts. Analyzing this feedback alongside behavioral data provides a comprehensive view of the user experience, allowing companies to address issues and prioritize enhancements.
5. Monitoring Performance and Load Times
Performance issues, such as slow load times or frequent crashes, can severely impact user experience. SaaS companies should use monitoring tools like Datadog to track performance metrics in real-time and identify any bottlenecks. Ensuring that the product runs smoothly and efficiently is essential for maintaining a positive user experience.
6. Segmenting Users for Targeted UX Improvements
Not all users have the same needs or expectations, so a one-size-fits-all approach to UX may not be effective. By segmenting users based on demographics, usage patterns, or subscription levels, SaaS companies can identify specific groups that may require targeted UX improvements. For example, new users might benefit from more guided onboarding, while power users might need advanced features or shortcuts.
7. Utilizing Predictive Analytics for Proactive UX Enhancements
Predictive analytics can help SaaS companies anticipate user needs and proactively enhance the user experience. By analyzing historical data and identifying patterns, companies can predict which features users are likely to need next, when they might face challenges, or when they might churn. This allows SaaS companies to address issues before they become problems, leading to a smoother and more satisfying user experience.
What Role Does Predictive Analytics Play in SaaS Growth?
Predictive analytics involves using historical data, machine learning, and statistical models to forecast future outcomes. For SaaS companies, predictive analytics can be a game-changer, driving growth by enabling proactive decision-making and optimization. Here’s how predictive analytics can fuel SaaS growth.
1. Anticipating Customer Behavior and Churn
One of the most powerful applications of predictive analytics in SaaS is anticipating customer behavior, including the likelihood of churn. By analyzing patterns in user activity, engagement, and support interactions, predictive models can identify customers who are at risk of canceling their subscriptions. SaaS companies can then take proactive measures—such as offering personalized support, discounts, or feature enhancements—to retain these customers and reduce churn.
2. Enhancing Customer Lifetime Value (CLTV)
Predictive analytics can help SaaS companies maximize CLTV by identifying opportunities for upselling, cross-selling, and renewing subscriptions. By analyzing customer data, SaaS companies can predict which customers are most likely to upgrade to higher-tier plans, purchase additional features, or renew their subscriptions. Targeted marketing and sales efforts based on these predictions can significantly boost revenue.
3. Optimizing Marketing Campaigns
Predictive analytics can also enhance the effectiveness of marketing campaigns by identifying the most promising leads and channels. By analyzing past campaign data, SaaS companies can predict which prospects are most likely to convert, which marketing messages resonate best, and which channels offer the highest ROI. This allows for more focused and cost-effective marketing strategies, driving higher acquisition rates at lower costs.
4. Improving Product Development
Predictive analytics can inform product development by forecasting which features will be most in demand, which enhancements will have the biggest impact on user satisfaction, and which pain points need to be addressed. By incorporating predictive insights into the product roadmap, SaaS companies can prioritize development efforts that align with customer needs and market trends, leading to faster growth and stronger product-market fit.
5. Forecasting Revenue and Growth
Accurate revenue forecasting is critical for SaaS companies to make informed decisions about scaling, investment, and resource allocation. Predictive analytics can provide more accurate revenue forecasts by analyzing historical sales data, market trends, and customer behavior. These forecasts can help SaaS companies plan for growth, manage cash flow, and set realistic financial goals.
6. Enhancing Customer Support
Predictive analytics can also improve customer support by anticipating common issues and optimizing resource allocation. For example, by analyzing support ticket data, SaaS companies can predict when and where spikes in support requests are likely to occur, enabling them to staff appropriately and resolve issues faster. Predictive models can also suggest solutions to common problems, allowing support teams to address issues more efficiently.
7. Driving Data-Driven Decision-Making
At its core, predictive analytics empowers SaaS companies to make data-driven decisions that are proactive rather than reactive. By leveraging predictive insights, SaaS companies can anticipate challenges, capitalize on opportunities, and stay ahead of the competition. This forward-thinking approach is essential for sustained growth in the competitive SaaS landscape.
How Can Data Analytics Help in Optimizing SaaS Pricing?
Pricing is one of the most critical decisions for any SaaS company, directly impacting revenue, customer acquisition, and retention. Data analytics can play a pivotal role in optimizing SaaS pricing by providing insights into customer behavior, willingness to pay, and market trends. Here’s how SaaS companies can use data analytics to fine-tune their pricing strategies.
1. Understanding Customer Segments and Willingness to Pay
Data analytics allows SaaS companies to segment their customer base based on factors like usage patterns, company size, industry, and value received from the product. By analyzing these segments, companies can identify different willingness-to-pay levels and tailor pricing models accordingly. For example, enterprise customers may be willing to pay more for advanced features and dedicated support, while small businesses might prefer a lower-priced, more basic plan.
2. Analyzing Competitor Pricing
Competitor analysis is a crucial aspect of pricing strategy. By using data analytics to monitor competitors’ pricing models, discounts, and promotional strategies, SaaS companies can position themselves more effectively in the market. Analytics can reveal pricing gaps, allowing companies to adjust their prices to be more competitive or to differentiate themselves based on value.
3. Testing and Refining Pricing Models
A/B testing can be applied to pricing just as it is to other aspects of product development. SaaS companies can experiment with different pricing models—such as tiered pricing, usage-based pricing, or freemium models—and use data analytics to assess their impact on conversion rates, customer satisfaction, and revenue. Over time, this iterative approach helps identify the pricing model that maximizes profitability while meeting customer needs.
4. Dynamic Pricing Based on Usage and Demand
Dynamic pricing involves adjusting prices based on real-time data, such as usage patterns, demand fluctuations, or customer behavior. SaaS companies can use data analytics to implement dynamic pricing strategies that respond to changes in customer needs or market conditions. For example, a SaaS company might offer lower prices during periods of low demand or introduce surge pricing for premium features during peak usage times.
5. Predicting the Impact of Price Changes
Before implementing price changes, SaaS companies can use predictive analytics to forecast their potential impact on revenue, customer acquisition, and churn. By analyzing historical data and simulating different pricing scenarios, companies can make more informed decisions about whether to increase, decrease, or restructure their pricing. This reduces the risk of negative consequences, such as losing customers due to a price hike.
6. Optimizing Discount and Promotion Strategies
Discounts and promotions are common tools for driving customer acquisition and retention, but they must be used strategically to avoid eroding long-term value. Data analytics can help SaaS companies optimize their discount strategies by identifying which types of promotions resonate most with different customer segments and which timing and frequency yield the best results. This ensures that discounts drive growth without undermining profitability.
7. Aligning Pricing with Customer Value
Ultimately, the most successful pricing strategies align closely with the value that customers perceive in the product. Data analytics allows SaaS companies to continuously assess the relationship between pricing and perceived value, ensuring that customers feel they are getting a fair deal. By regularly analyzing customer feedback, usage data, and market trends, SaaS companies can adjust their pricing to reflect the evolving value of their product.
What Are Some Advanced Data Analytics Techniques for SaaS?
As SaaS companies mature in their data analytics capabilities, they can leverage more advanced techniques to gain deeper insights and drive further growth. Here are some advanced data analytics techniques that SaaS companies can explore.
1. Cohort Analysis
Cohort analysis involves grouping users based on shared characteristics or behaviors and tracking their performance over time. For example, a SaaS company might group users who signed up during a specific month or who use a particular feature. By analyzing these cohorts, companies can identify trends, such as how long it takes for users to reach certain milestones, which cohorts have the highest retention rates, or how product changes affect different groups.
2. Predictive Modeling
Predictive modeling uses machine learning algorithms to forecast future outcomes based on historical data. SaaS companies can use predictive models to anticipate customer behavior, such as churn risk, upsell opportunities, or the likelihood of conversion. These models enable proactive decision-making, allowing companies to address issues before they arise and capitalize on potential opportunities.
3. Customer Segmentation with Clustering Algorithms
Clustering algorithms, such as K-means clustering, can be used to automatically segment customers into groups based on similarities in their data. This advanced technique helps SaaS companies identify distinct customer segments that may not be immediately obvious. By understanding these segments, companies can tailor their marketing, sales, and product strategies to meet the specific needs of each group.
4. Time Series Analysis
Time series analysis involves analyzing data points collected or recorded at specific time intervals. For SaaS companies, this can be used to track trends in metrics such as MRR, churn rate, or user engagement over time. Time series analysis can reveal patterns, seasonal variations, or anomalies, helping companies make more informed decisions about forecasting and resource allocation.
5. Sentiment Analysis
Sentiment analysis uses natural language processing (NLP) to analyze customer feedback, such as reviews, surveys, or social media posts, to determine the overall sentiment—whether positive, negative, or neutral. SaaS companies can use sentiment analysis to gauge customer satisfaction, identify common pain points, and respond to customer needs more effectively.
6. Behavioral Segmentation
Behavioral segmentation involves dividing users into groups based on their interactions with the product. This could include factors like frequency of use, feature adoption, or support interactions. By understanding these behaviors, SaaS companies can tailor their product development, marketing, and customer success strategies to better meet the needs of different user segments.
7. Anomaly Detection
Anomaly detection uses machine learning to identify unusual patterns or outliers in data that may indicate a problem, such as sudden spikes in churn, unexpected drops in user engagement, or security breaches. SaaS companies can use anomaly detection to quickly identify and address issues before they escalate, ensuring a stable and reliable product experience.
Your Turn…
Data analytics is not just a tool for SaaS companies—it’s the backbone of modern SaaS success. By tracking key metrics, fostering a data-driven culture, and using the right tools, SaaS companies can gain deep insights into their business, optimize their strategies, and drive sustainable growth. From improving user experience to optimizing pricing and predicting future trends, the power of data analytics is immense.
SaaS companies that embrace data-driven decision-making will be better equipped to navigate the challenges of a competitive market, meet customer needs, and achieve long-term success. Whether you’re just starting to explore data analytics or looking to refine your existing strategies, the key is to remain agile, continuously learn from your data, and never stop seeking new opportunities to leverage insights for growth.
For more insights into the future of AI in SaaS, subscribe to our newsletter and stay updated with the latest trends and developments.